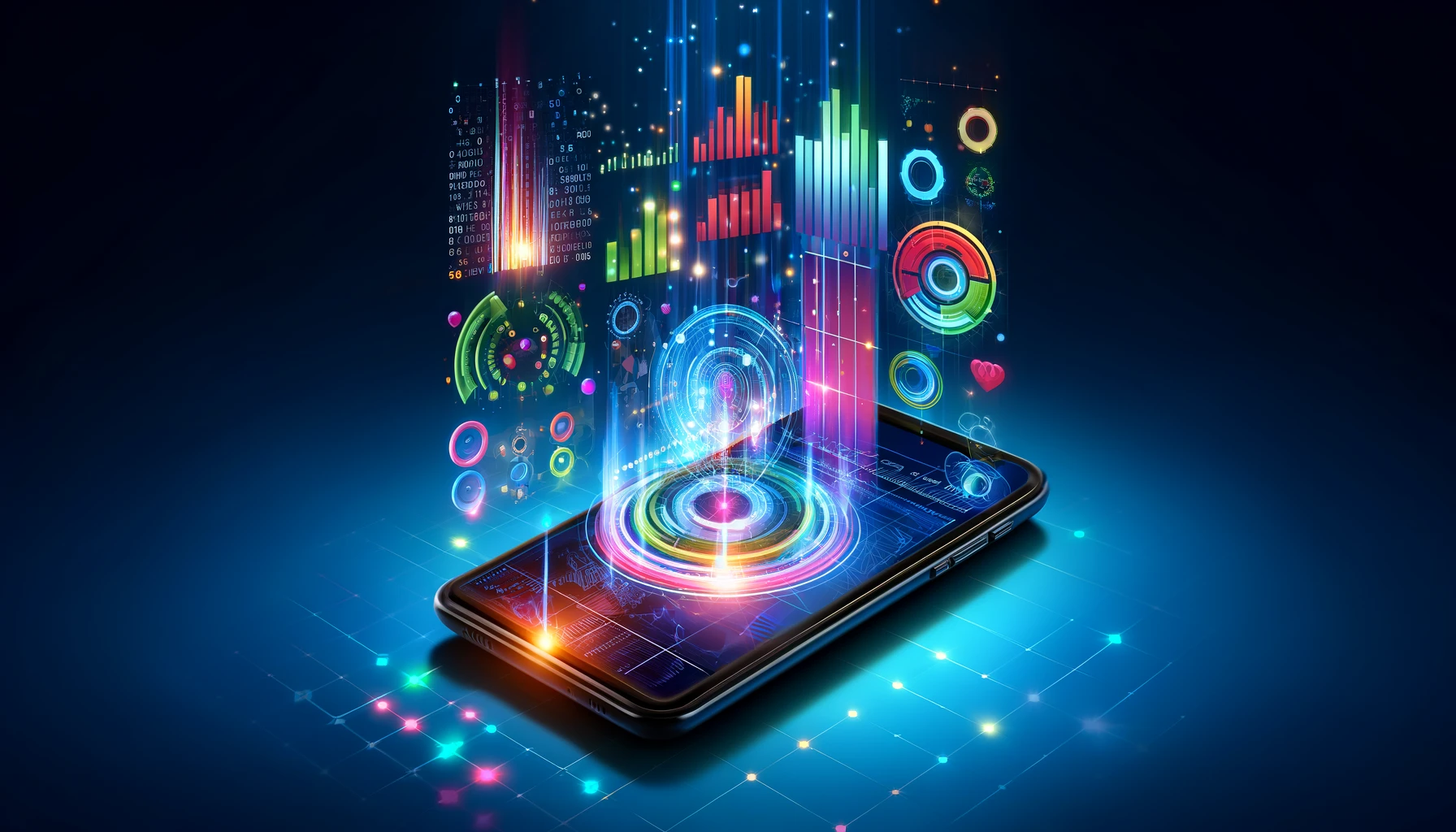
The rapid pace of change in mobile marketing requires innovative strategies to anticipate and meet user needs. Predictive analytics has emerged as a powerful tool, enabling marketers to forecast behavior, improve personalization, and drive growth. By leveraging predictive data insights, businesses can optimize their marketing campaigns and stay ahead of competitors. This article dives into the core concepts and practical applications of predictive analytics in mobile marketing.
What is Predictive Analytics?
Predictive analytics involves analyzing historical and real-time data to forecast future events, trends, and user behaviors. Advanced machine learning algorithms identify patterns that help marketers predict outcomes such as user churn, engagement rates, and purchasing behavior.
Key Benefits of Predictive Analytics in Mobile Marketing:
- User Segmentation and Personalization: Predictive analytics identifies clusters of users with similar characteristics and behaviors. Marketers can create highly personalized campaigns tailored to each segment, resulting in improved engagement and conversion.
- Churn Reduction: By analyzing engagement data and user activity, predictive models identify potential churn candidates early. Marketers can then implement retention strategies like targeted discounts, personalized messages, and exclusive offers to keep users engaged.
- Optimal Timing for Notifications:Predictive tools analyze when users are most likely to interact with the app, allowing marketers to send notifications and messages at times when users are most receptive, increasing open rates and conversions.
- Lifetime Value (LTV) Prediction:By evaluating historical behavior, predictive models estimate the future value of each user. This insight allows marketers to allocate resources more efficiently, focusing on acquiring and retaining high-value users.
- Content Recommendations: Analyzing previous engagement with different content types enables predictive models to recommend content that will resonate most with individual users, boosting session lengths and satisfaction.
Steps to Implement Predictive Analytics:
1. Setting Clear Objectives
- What: Begin by defining what you aim to achieve with predictive analytics. Is it reducing churn rates, increasing engagement, or driving more in-app purchases?
- How: Collaborate with marketing, data, and product teams to clarify objectives.
- Break down goals into measurable metrics like CLV, retention rates, or conversion rates.
2. Gathering and Integrating Data
- What: Ensure you have comprehensive, high-quality data to build reliable predictive models.
- How: Aggregate information from various sources like app usage, transactions, support tickets, and external platforms.
- Clean and normalize data to eliminate inconsistencies and errors.
- Seamlessly integrate data with your chosen predictive analytics tool.
3. Choosing the Right Predictive Analytics Tool
- What: Find a platform that matches your marketing needs, data requirements, and budget.
- How: Assess different tools based on features, scalability, and integration with existing systems.
- Run pilot tests to compare insights, user interface, and ease of implementation.
- Prioritize platforms that offer pre-built integrations with your current data ecosystem.
4. Building and Training Predictive Models
- What: Develop models that accurately forecast user behavior to meet your goals.
- How: Start with templates or pre-existing models offered by the platform, if available.
- Train models using historical data, enabling algorithms to detect trends and patterns.
- Refine feature selection to ensure the models focus on the most relevant data points.
5. Deploying Models and Automating Actions
- What: Use predictive models to automate personalized marketing actions.
- How: Link predictive models to marketing automation systems.
- Define criteria for triggering actions, like user retention campaigns or targeted notifications.
- Automate tailored responses, such as push notifications or special offers, based on predictive scores.
6. Monitoring, Evaluating, and Refining Models
- What: Ensure models remain accurate by continuously monitoring and refining them.
- How: Monitor model performance using metrics like precision, recall, and lift.
- Regularly review trends in user behavior to adapt to changing conditions.
- Update models by retraining with fresh data and adjusting prediction thresholds.
7. Scaling and Optimizing Marketing Strategies
- What: Use insights from predictive analytics to refine and scale your marketing efforts.
- How: Identify successful predictive marketing strategies to apply to other campaigns or segments.
- Experiment through A/B testing for optimal timing, messaging, and audience segmentation.
- Adapt marketing tactics continually based on insights gained through predictive models.
By following this structured approach, you’ll gain a deeper understanding of your user base, allowing you to anticipate their needs and refine your mobile marketing strategies. Predictive analytics will empower your business to stay ahead of the competition and achieve sustainable growth.
Tools for Implementing Predictive Analytics in Mobile Marketing
-
- Overview: Firebase provides robust analytics capabilities designed specifically for mobile apps. It offers integration with Google’s machine learning platform, allowing you to predict user behavior based on app usage patterns.
-
- Features: Event tracking, user property collection, audience segmentation, and integration with other Firebase features like Cloud Messaging and Remote Config.
-
- Overview: CleverTap combines real-time user insights, an advanced segmentation engine, and powerful engagement tools to deliver personalized mobile marketing.
-
- Features: Predictive segmentation based on user actions and inactivity, automated campaigns, and A/B testing.
-
- Overview: Known for its comprehensive analytical capabilities, Adobe Analytics offers deep insights into user paths within apps, helping predict future behaviors.
-
- Features: Flow analysis, cohort analysis, and predictive scoring.
-
- Overview: Mixpanel excels in user interaction tracking and provides predictive analytics to understand likely user actions before they happen.
-
- Features: Retention analysis, funnel visualization, and user journey analytics.
-
- Overview: Amplitude focuses on product intelligence and behavior-driven growth. Its predictive capabilities help identify trends and user segments likely to convert or churn.
-
- Features: Advanced user segmentation, real-time analytics, and prediction models.
Final Thoughts
Predictive analytics equips mobile marketers with valuable insights into user behavior, enabling them to address needs and personalize marketing efforts proactively. By integrating this technology into their marketing strategies, app developers can deliver better user experiences, reduce churn, and improve overall ROI. Staying ahead of the competition requires reacting to current trends, predicting future opportunities, and adapting in real-time. Predictive analytics makes this possible, empowering businesses to thrive in a highly competitive mobile landscape.